Tutor HuntResources Engineering Resources
Automated Particle Recognition For Engine Soot Based On Machine Learning Algorithms
Dissertation project abstract
Date : 20/11/2020
Author Information
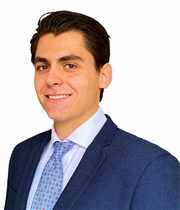
Uploaded by : Leonardo
Uploaded on : 20/11/2020
Subject : Engineering
Characterization of soot nanoparticle morphology can be used to better understand the effects on human health and the contribution to the greenhouse effect and climate change. Developed in previous work by our research group, 3D Transmission Electron Microscopy (3D-TEM) is a state-of-art technology to reconstruct soot nanoparticles into 3D computational models. While 3D-TEM provides details about the topographic structure of soot that are not easily discernible in a typical 2D representation of soot, the technology is expensive to run due to the time required to construct the representations and to manually detect soot nanoparticles on the TEM sample grid. In this work, machine learning techniques were applied to contribute towards making 3D TEM fully automated and more efficient to use compared to traditional 2D-TEM, by replacing the manual soot detection with Convolutional Neural Networks. ResNet18 and ResNet50, pre-trained Convolutional Neural Network (CNN) architectures, were trained to detect soot nanoparticles in montages representing the oil samples studied. 7 tests were conducted to determine how using a deeper network affects accuracy, achieving the greatest accuracy of 91.6% when 50 images of soot and 50 images of non-soot were learned by ResNet18 architecture, resulting in a decrease in performance when the deeper network Resnet50 was tested. Resnet18 is therefore used to evaluate the final software it is trained on 5 datasets with an increasing number of soot images to investigate whether testing accuracy on a montage of 228 images increased by increasing training set. The accuracy increased when the number of training images was greater, showing that performance parameters improved by increasing training dataset, reaching the greatest overall detection accuracy of 90%. One image was classified in 0.132 seconds when the automated procedure was used, while an image was manually classified in around 1.2 seconds. This implied a time reduction of a magnitude of 9, making the software beneficial in particular when large amounts of images are processed, saving just under 35.6 minutes when 2,000 images tested (10 montages). As cost of labor is very high, removing the need of a human operator truly contributes increasing the procedure s efficiency. The work shows that pre-trained CNN s can be used with TEM images, opening a range of opportunities to apply the technology in nanoparticles research.
This resource was uploaded by: Leonardo